About
Wake Vision is a state-of-the-art person detection dataset specifically created for TinyML applications. It provides a comprehensive collection of high-quality images and precise annotations to train and evaluate machine learning models for efficient person detection on embedded and edge devices. Wake Vision also includes a fine-grain benchmark suite for evaluating the robustness of TinyML models.
The Dataset
Wake Vision is a large, high-quality binary image classifcation dataset for person detection:
- Over 6 million high-quality images
- Two training sets (Large & Quality)
- High quality validation and test sets (~2% Label Error Rate)
Fine-Grain Benchmark Suite
Wake Vision also incorporates a comprehensive fine-grained benchmark to assess fairness and robustness across:
- Perceived gender
- Perceived age
- Subject distance
- Lighting conditions
- Depictions (e.g., drawings, digital renderings)
Access The Dataset
Key Features
TinyML Focus
TinyML relevant usescase and tractable task.
Two Training Sets
One large and one high quality, ideal for data-centric AI research
Diverse Scenarios
Wide range of person detection use cases
High-Quality Test and Val
Manually labeled to ensure reliable evaluation
Leaderboard
Model Name | Input Size | RAM (KiB) | Flash (KiB) | MACs | Test Accuracy |
---|---|---|---|---|---|
mcunet-vww2 | (144,144,3) | 393 | 923.76 | 56,022,934 | 85.6±0.34% |
MobileNetV2_0.25 | (224,224,3) | 1,244.5 | 410.55 | 36,453,732 | 84.9±0.11% |
mcunet-vww1 | (80,80,3) | 226.5 | 624.55 | 11,645,502 | 82.9±0.29% |
mcunet-vww0 | (64,64,3) | 168.5 | 533.84 | 5,998,334 | 81.7±0.28% |
micronet_vww4 | (128,128,1) | 123.50 | 417.03 | 18,963,302 | 77.9±0.6% |
micronet_vww3 | (128,128,1) | 137.50 | 463.73 | 22,690,291 | 77.8±0.56% |
colabnas_k_8 | (50,50,3) | 32.5 | 44.56 | 2,135,476 | 77.3±0.37% |
colabnas_k_4 | (50,50,3) | 22 | 18.49 | 688,790 | 75.7±0.18% |
micronet_vww2 | (50,50,1) | 71.50 | 225.54 | 3,167,382 | 71.9±0.67% |
colabnas_k_2 | (50,50,3) | 18.5 | 7.66 | 250,256 | 70.6±0.96% |
ymac | (50,50,3) | 34.5 | 27.77 | 431,985 | 76.7±0.51% |
samy | (80,80,3) | 73.5 | 34.55 | 5,718,046 | 79.5±0.61% |
anas-benalla | (50,50,3) | 48.5 | 104.55 | 4,899,292 | 79.7±0.28% |
mohammad_hallaq | (80,80,3) | 129.5 | 128.32 | 3,887,331 | 77.3±0.5% |
apighetti | (50,50,3) | 48.5 | 278.36 | 693,818 | 74.3±0.22% |
benx13 | (48,48,3) | 63.5 | 94.95 | 1,693,474 | 79.6±0.22% |
cezar | (50,50,3) | 245.5 | 57.23 | 20,435,868 | 79.0±0.42% |
🙋♂️ Contribute
Share your results with us and contribute to the leaderboard, or you can issue a PR at this link!
🏆 Challenge
The first edition of the Wake Vision Challenge has ended. Stay tuned for the next edition!
Wake Vision Challenge 🏆
Following the release of the Wake Vision Dataset, the Wake Vision Challenge was launched to advance research in TinyML. Participants were invited to contribute innovative model architectures in the model-centric track or to improve the dataset through the data-centric track. This section reports the results of the challenge. By clicking on the author name visitors can access the submitted model, source code, and a report describing the adopted solution. This provides a foundation for those interested in pushing the boundaries of TinyML with the Wake Vision Dataset.
Model-Centric Track
Author | Model Name [.tflite] | Flash [B] | RAM [B] | MACs | Deployability | Test Acc. | Norm. Test Acc. | Score | |
---|---|---|---|---|---|---|---|---|---|
1° | ymac | wv_k_8_c_5_sepconv | 9236 | 20492 | 431985 | 1.0 | 0.726798288002209 | 0.8469608145634063 | 0.8633991440011045 |
2° | samy | wv_k_8_c_5_80_small | 25584 | 23424 | 5718046 | 0.885452561091946 | 0.7678862349855032 | 1.0 | 0.8266693980387246 |
3° | anas-benalla | model_5K | 52712 | 24808 | 4899292 | 0.8624280319006686 | 0.7644622394035621 | 0.9872467345469506 | 0.8134451356521153 |
4° | mohammad_hallaq | wv_k_8_c_5_v4 | 55392 | 61968 | 3887331 | 0.7986097171762788 | 0.7525058677343642 | 0.9427131543762214 | 0.7755577924553215 |
5° | apighetti | quant_aaaabh | 276872 | 34480 | 693818 | 0.6331896220580955 | 0.743642137235952 | 0.9096986526792142 | 0.6884158796470237 |
6° | benx13 | mcunet_tiny_int8 | 42980 | 48680 | 1751060 | 0.8773231889307195 | 0.49940632334667956 | 0.0 | 0.6883647561386995 |
7° | cezar | bestmodel | 24840 | 180644 | 20435868 | 0.31389897721781335 | 0.7530719315200883 | 0.9448215571325722 | 0.5334854543689509 |
Data-Centric Track
Author | Model Name | Test Acc. | |
---|---|---|---|
1° | kooks | wv_quality_mcunet-320kb-1mb_vww | 0.7915366560817341 |
2° | rgroh | wv_quality_mcunet-320kb-1mb_vww | 0.7672925583321828 |
3° | benx13 | mcunet_tiny_int8 | 0.4954576832804087 |
Example Images
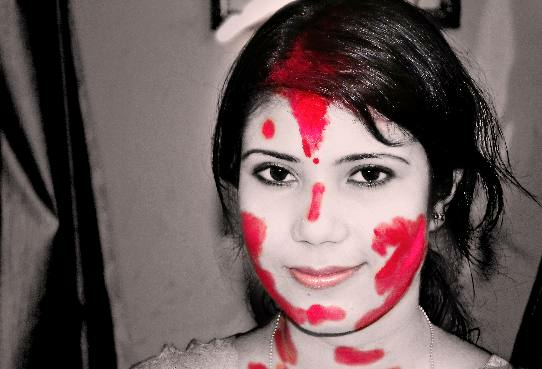
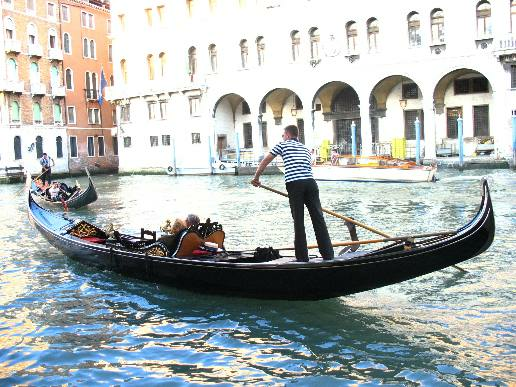
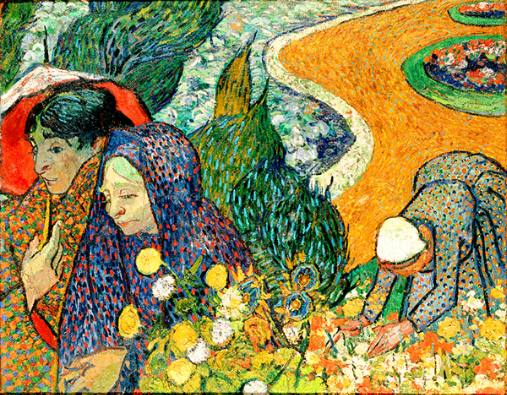
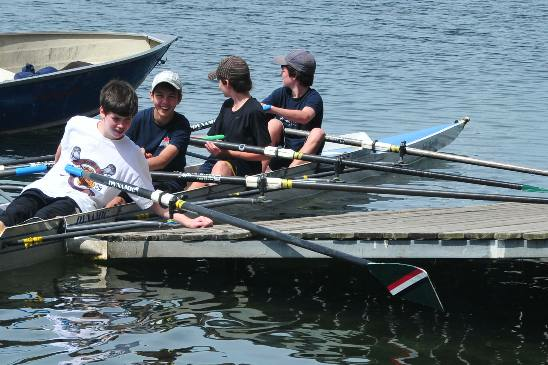
License
The Wake Vision labels are derived from Open Image's annotations which are licensed by Google LLC under CC BY 4.0 license. The images are listed as having a CC BY 2.0 license. Note from Open Images: "while we tried to identify images that are licensed under a Creative Commons Attribution license, we make no representations or warranties regarding the license status of each image and you should verify the license for each image yourself."
Cite
title={Wake Vision: A Tailored Dataset and Benchmark Suite for TinyML Computer Vision Applications},
author={Banbury, Colby and Njor, Emil and Garavagno, Andrea Mattia and Stewart, Matthew and Warden, Pete and Kudlur, Manjunath and Jeffries, Nat and Fafoutis, Xenofon and Reddi, Vijay Janapa},
journal={arXiv preprint arXiv:2405.00892},
year={2024}
}
Contact
Email: emjn@dtu.dk cbanbury@g.harvard.edu AndreaMattia.Garavagno@edu.unige.it
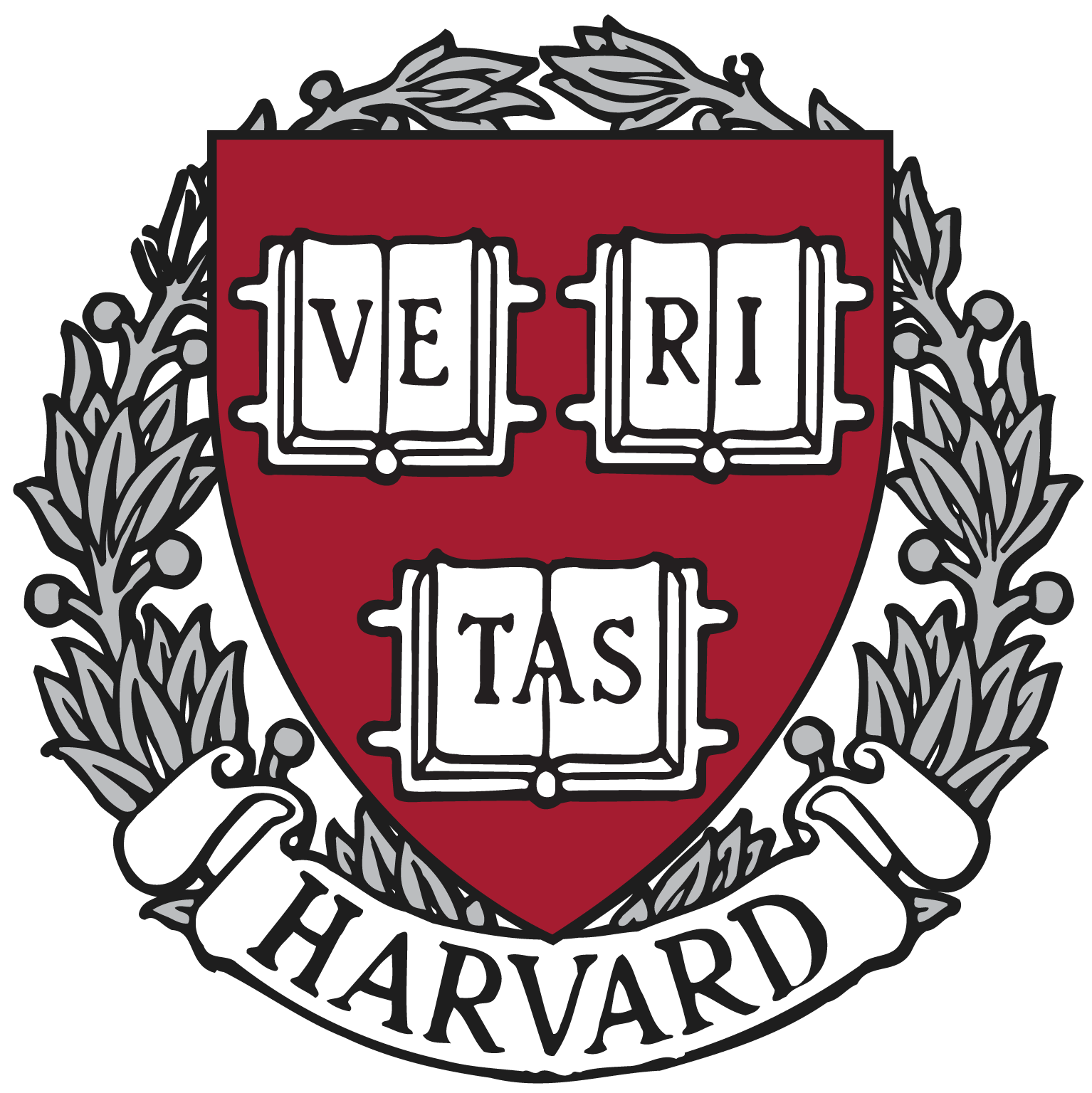